Searching for the facts in a global pandemic
In times of great uncertainty, such as global pandemics, the appetite for reliable and trustworthy information increases. We look to official authorities and our peers for guidance. However, as has quickly become evident, the veracity and authenticity of information being circulated is not always sound. As people are increasingly connected to their devices, checking social media and news sites for information and guidance to inform their own actions, the potential impact of misleading and harmful content is more keenly observed.
Misinformation exacerbates uncertainty and provokes emotive responses such as fear, anger and distrust. Furthermore, it can also inform potentially harmful behaviours and attitudes, which in the context of a global health pandemic, carry high stakes. In the context of COVID-19, the global reach and severity of the situation, together with the increased connectivity afforded by digital platforms, make for a dangerous combination.
The World Health Organisation (WHO) explain that the ‘infodemic’ – the ‘over-abundance of information – some accurate and some not’ – is a significant concern, and ‘makes it hard for people to find trustworthy sources and reliable guidance when they need it’.
World Health Organisation
How are platforms dealing with misleading content?
Social media platforms such as Twitter have reported a surge in usage since the onset of the pandemic, making for a particularly captive audience for information, whether accurate or not. The director-general of the World Health Organization (WHO), Tedros Adhanom Ghebreyesus, warned in his address on 15 February 2020 that ‘[f]ake news spreads faster and more easily than this virus, and is just as dangerous’.
In a statement released on 11 May 2020, Twitter outlined their updated guidelines on how they are handling misleading content about COVID-19 on their platform. They have begun using a tiered approach to determine how problematic content should be handled. Content is to be classified based on potential harm (moderate/severe) and whether it is misleading, disputed or unverified.
Misleading content, i.e. a tweet that includes statements that have been confirmed false, where the propensity for harm is severe, will be removed. For moderate harm the tweet will remain but be accompanied by a label, reading Get the facts about COVID-19, linking trusted information sources or additional information about the claim. For tweets containing information about disputed claims that carry potential for severe harm, a warning will be issued, and the user will have to choose to reveal the tweet.
What isn’t clear is exactly how Twitter are carrying out these fact-checks. In an earlier blog post, Twitter stated that they were stepping up their automatic fact-checking in response to COVID-19. Fact-checking is an increasingly vital approach for tackling the rapid spread of false claims online. While there is an urgent need for automated systems that detect, extract and classify incorrect information in real time, it is an extremely challenging task.
Why automated fact-checking is so difficult
In January we held a fact-checking hackathon (you can read more about that here) where participants developed automated approaches to assess claims made about wikipedia data. Even with a static set of claims and predefined evidence sources, the process of verifying claims as true or false is highly complex. To start with, you need a veracious baseline to assess against, a database of facts and falsehoods. This is challenging in the current context, when new information is constantly being generated. The International Fact Checking Network (IFCN) has created a database of fact-checks, sourced from fact-checking organisations across the world. However, while this is an extremely useful resource it is not a complete database. Then, given a claim to be fact-checked, and the availability of a corresponding fact-checked entry in your veracious database, you need to classify the claim sentences either as supporting or refuting the original claim or else as providing too little information to either support or refute it. If we consider the following example:
“5G is responsible for COVID-19.“
This certainly reads as a pretty unequivocal endorsement for the (debunked) theory that 5g is linked to how COVID-19 spreads.
However, what if we consider the following:
“Oh okay… 5G is responsible for COVID-19. Come on people!“
For a human reader, the preceding `oh okay’, and tailing `come on people!’, suggests that this could be an incredulous response to the proposed link between the virus and mobile internet connectivity. Language is flexible and interpreting the implied meaning often requires us to consider context, both what is said around a particular sentence, but also the interactional sequence in which it occurs, for example:
“What is the most ridiculous conspiracy theory about COVID-19 you have heard?“
Reply: “5G is responsible for COVID-19.“
While this example served to emphasise how relatively straightforward sentences can be taken out of context, content designed to mislead often strategically incorporates truth or half-truths into the content. As Kate Starbird (2019) observed, ‘disinformation often layers true information with false — an accurate fact set in misleading context, a real photograph purposely mislabelled’ (Kate Starbird, 2019). The recently published Reuters Institute factsheet summarising COVID-19 misinformation, corroborates this: 87% of the misinformation in their sample circulated on social media involved `various forms of reconfiguration, where existing and often true information is spun, twisted, recontextualised, or reworked’, while, purely fabricated content accounted for only 12%.
It is easy to see how content can easily be misclassified, and this is a key challenge for automated fact-checking. If systems are oversensitive or insufficiently trained then content can be incorrectly flagged and removed. In March, Facebook’s efforts to control the spread of misinformation led to genuine news articles to removed from its site. Twitter acknowledge this limitation and explain that they will be cautious in their approach and are unlikely to immediately suspend accounts:
“We want to be clear: while we work to ensure our systems are consistent, they can sometimes lack the context that our teams bring, and this may result in us making mistakes. As a result, we will not permanently suspend any accounts based solely on our automated enforcement systems.”
Twitter blog post
By the time a post is flagged and removed it will likely already have been seen by many people. Ideas are very resilient and hard to unpick, particularly when they are shared at speed and integrated into individuals’ sense-making practices. A recent article in the New York Times showed that in little over 24 hours a misleading Medium article was read by more than two million people and shared in over 15 thousand tweets, before the blog post was removed.
Consequently, fact-checking approaches can only be so effective. Even if misleading content is flagged or reported, will it be removed? And if it is, by that time it will have spread many times over, been reformulated and shared in other forms that may fly under the radar of detection algorithms.
Fact-checking alone is not enough
Even if a perfect automated fact-checking system did exist, the problem still remains, which is why empirical evaluation of strategies such as those being rolled out by social media platforms needs to be carried out.
While disinformation campaigns are typically thought to be powered by nefarious actors, misinformation can be passed on by well-meaning individuals who think they are sharing useful and newsworthy information. A key challenge is that once a piece of fake news has made an impression it can be challenging to correct. Furthermore, it has been shown that some people are more resistant to fact-checking than others, and even once a claim has been disproved, biased attitudes remain (De keersmaecker & Roets, 2017).
A paper published by Vosoughi et al (2018) found that “false news spreads more pervasively than the truth online”. This is particularly problematic, as one way we judge something to be true or false is by how often we come across it (exposure bias). Furthermore, fake news is typically created to be memorable and newsworthy; with emotive headlines that appeal to the concerns and interests of its readership, playing on the reader’s emotional biases and tapping into their pre-existing attitudes (myside bias). Considering the cognitive processes that impact on how individuals engage with misinformation can inform new thinking on how we can mitigate its effects. Recent work by Rozenbeek & Van der Linden highlights psychological inoculation can develop attitudinal resistance to misinformation.
Slow but steady progress
Clearly, working out how best to approach these challenges is not straightforward, but it is promising that there is a concerted effort to develop resources, tools and datasets to assist in the pursuit. Initiatives, such as The Coronavirus Tech Handbook, a crowdsourced library of tools and resources related to COVID-19. Initiated by Newspeak House including a section on misinformation, highlight how distributed expertise can be harnessed and collated to support collective action.
Similarly, it is promising to see organisations experimenting with different formats for information provision, that may prove more accessible for people trying to navigate through the masses of information available. For example, the Poynter Institute’s International Fact-Checking Network (IFCN) has created a WhatsApp chatbot and the WHO has developed a chatbot for facebook. While these use very constrained interaction formats, i.e. choosing from preselected categories to access information, these may well be more accessible than reading a long web page for many.
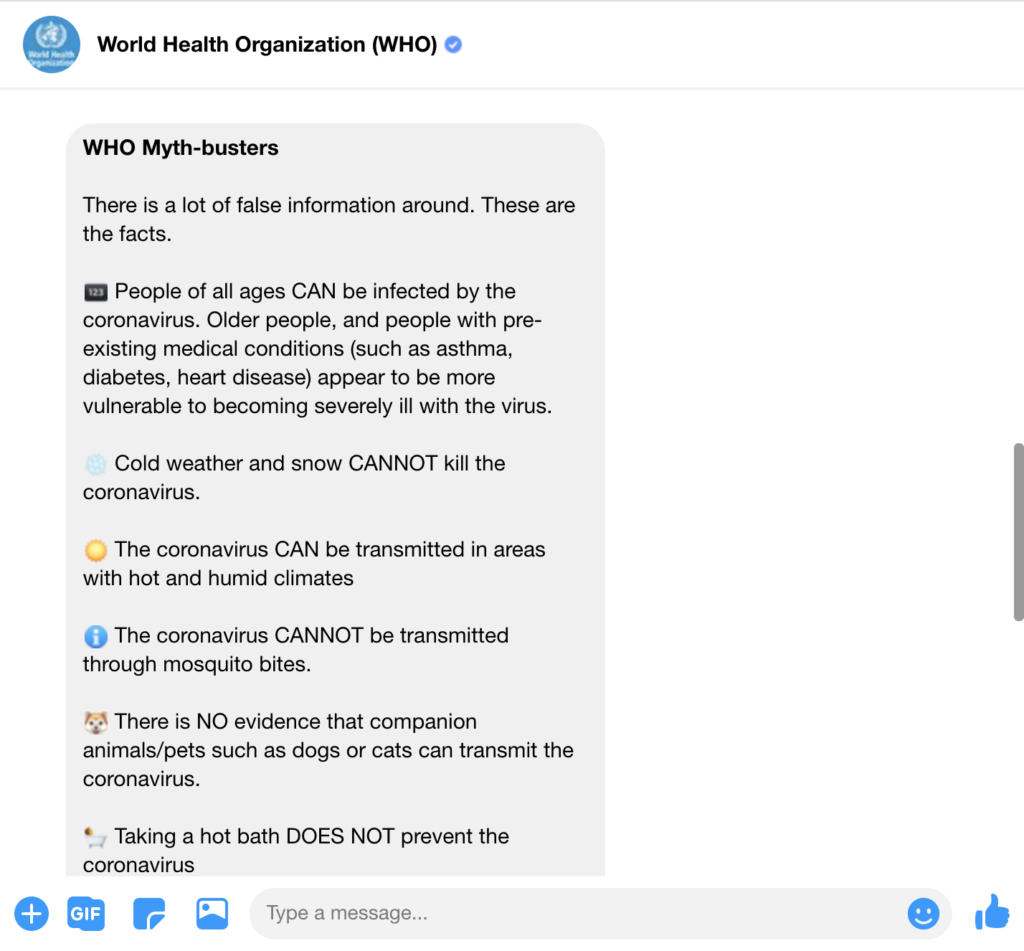
In the context of COVID-19, individuals are required to interpret what has at times been ambiguous guidance and make decisions about how best to protect themselves and their families. This backdrop of uncertainty will inevitably drive up information seeking behaviours. While it may seem that each day unveils new examples of misleading content, having pages dedicated to the issue on many leading websites can be no bad thing. Hopefully, it will help to build public awareness that assessing information online requires scrutiny. The issue comes down to trust and how we as content consumers assess which information we deem reliable.
In Spinning the Semantic Web, published over 17 years ago now, the understanding that a mechanism for encoding trust was essential to the flourishing of the internet was stated in the foreward by Tim Berners Lee. In this nascent projective vision of how the web should function, Lee explained, “statements of trust can be added in such a way as to reflect actual trust exactly. People learn to trust through experience and though [sic] recommendation. We change our minds about who to trust and for what purposes. The Web of trust must allow us to express this.” (Spinning the Semantic Web, p.xviii). He outlines the mission of the W3C to be to “help the community have a common language for expressing trust” not to take ”a central or controlling role in the content of the web.” Clearly the internet has developed in ways unforeseen by the authors in 2003, and in 2020 we find ourselves grappling with these very challenges of balancing top down and bottom up approaches to developing an ecosystem of trust.
Useful resources and further information
Full Fact, a UK based fact-checking organisation, has published a useful post on how individuals can guard themselves against misleading information https://fullfact.org/health/how-to-fact-check-coronavirus/.
If you would like to find out more about COVID-19 misinformation, we recommend the following podcasts:
Full Fact Podcast: https://play.acast.com/s/fullfactpodcast/a597558f-24a7-406e-aad8-330a09220520
Episode 5 of the Science and Policy podcast from the CSAP : http://www.csap.cam.ac.uk/Research-Policy-Engagement/science-and-policy-podcasts/
Nature CoronaPod – Troubling News: https://www.nature.com/articles/d41586-020-01137-7